Artificial intelligence (AI) systems can help physicians make clinical decisions and diagnoses. In the 1950s, Lipkin, Hardy, and Engle were the first to apply statistical methods to diagnose hematologic diseases (Engle et al., 1976). Then, with the refinement of studies and advancement in computer technology, artificial intelligence was created (Fashoto, 2015). Computer programs, known as clinical decision support systems (CDSSs) (Garg et al.), can analyze enormous volumes of complicated medical information to assist in clinical decision-making (Berner, 2007; Garg et al., 2005).
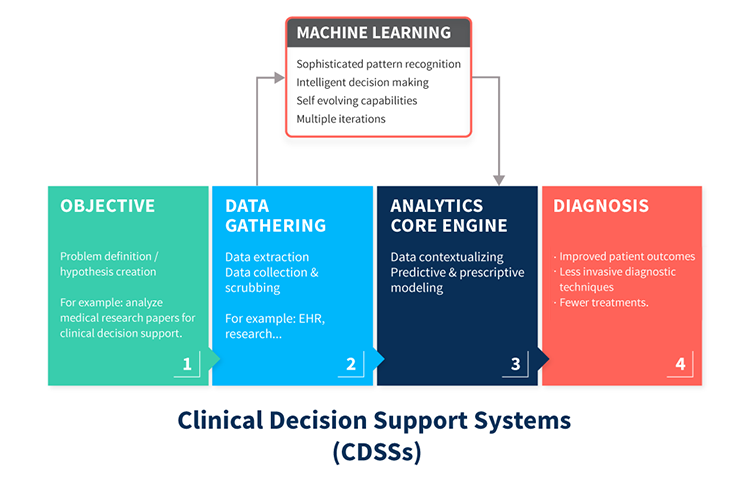
A recent example demonstrating the power of applied AI in CDSS in medicine relates to the story of a 60-year-old Japanese woman whose illness stumped doctors for months. A computer system, by the name of Watson, diagnosed the women’s illness as a rare form of leukemia in only 10 minutes after it examined millions of cancer research papers (Ng, 2016). Contrary to popular belief, AI has been capable of telling physicians what to do for a long time. Built in the 1970s, MYCIN was the first computer system providing clinical decision support to physicians that emulated human expert decision-making ability using artificial intelligence. Watson’s decrease in processing time is a significant change from that of MYCIN.
It is now hoped that with more powerful computing, quantum computing, that these CDSSs will increase efficiency and improve patient outcomes using less invasive diagnostic techniques and more directed treatments.
Classical Machine Learning (CML) is a subfield of artificial intelligence in which computer science derives patterns (that are learned) without being explicitly programmed. Computers can then make predictions by modeling raw input data that is stored and manipulated as small bits of information that exist as 0 or 1. For instance, with classical computing, information is stored and manipulated as small bits, whereas with quantum computing, information is stored and manipulated as quantum bits (qubits) that can exist in many states, known as superposition. This superposition (referred to as the quantum state) results in a substantial increase in the speed of complex computations by having the ability to compute in many states at once. Classical methods of machine learning are currently being adapted to quantum information processing in healthcare systems.
The healthcare reform act was passed in 2010 with the goals of improving the quality of medical care, reducing costs, and improving access to care, while broadening insurance coverage (Buntin, Jain, & Blumenthal, 2010). Quantum machine learning can benefit health care and assist in the implementation of healthcare reform with the improvement of patient care and reduction of costs for medical diagnostics and treatment. Arguments in support of using these machines in hospitals and laboratories include rapid diagnostic results, superior biomedical imaging capabilities, more precise cancer treatment or surgical procedures, and reminders/interventions for patient diagnostic tests or treatment schedules. Arguments against include the cost of hardware, the difficulty in deciphering diagnostic reasoning, and the potential for privacy or ethical issues.
The benefit to using quantum sensors and quantum imaging has far-reaching possibilities for the advancement of medicine. This technology could create novel techniques to evaluate the heart through magnetic fields and through more powerful imaging techniques. And, quantum computers will be able to process data much more quickly than traditional computers, which is especially useful for large images created by computed tomography (CT) or magnetic resonance imaging. Cancer research would be another area to benefit from the power of quantum computing. An increase in data transferring speed from lab to bedside would significantly improve the clinician’s ability to treat patients in an appropriate and timely manner (Jain & Chaturvedi, 2014).
Several research and internet articles have been published supporting the benefits of quantum computing in healthcare. CDSSs and quantum learning have shown great promise is in biomedical imaging. Enhanced imaging would allow for earlier detection of molecular changes in the body, resulting in more treatment options for patients afflicted with diseases such as cancer or dementia, and subsequently improved outcomes.
Neural networks in classical machine learning have proved to be an accurate diagnostic technique, optimized by changes in the parameters of the network’s architecture. These optimization methods are best suited for quantum computing, where the ability of “quantum tunneling” allows for optimization problems to be solved quickly. One study used a quantum-based binary neural network for the diagnosis of liver disease. This quantum-based algorithm was more accurate and precise in diagnosing liver disease when compared to a classical neural network (O. Patel & Tiawari, 2015).
Emmanuel Candes, an applied mathematician at Stanford University, realized the real need for quantum computers when he worked in collaboration with other radiologists to develop dynamic magnetic resonance imaging (MRI) to record a patient’s heartbeat in real-time. This system required the use of advanced algorithms to create high-resolution videos from only a few MRI images. However, he realized that there was not enough memory in his computer to perform the analysis (Ouelette, 2013). Quantum computing’s distributed optimization approach handles problems such as what Candes encountered, and does so in a fraction of the time, that is, assuming you have enough Q-RAM. It was once thought that one could only store and process this much information by using massive parallel computing. Compared to random access memory (RAM), which uses n bits to randomly address N=2^n distinct memory cells, quantum random access memory (qRAM) uses n qubits to address any quantum superposition of N memory cells, resulting in a more robust qRAM algorithm (Gioovannetti, Lloyd, & Maccone, 2008). For example, by using a quantum algorithm that has Q-RAM and a small 70-qubit quantum processor the genome of every person on Earth could be searched for common patterns among different genes, all while protecting people’s privacy. The quantum-computing technician would only have access to a very small fraction of the total data and the analysis would be done quickly. These large databases may someday exist, as human genome sequencing becomes less expensive and businesses that sell genotyping services become more prevalent (Rebentrost, Mohseni, & Lloyd, 2013).
Pharmacists could also benefit from CDSSs and quantum learning in that there are over 100,000 different types of drug interactions. These systems alert pharmacists to drug interactions. Although one group found no difference between pharmacists receiving computerized alerts and those not receiving alerts, an overall potential to reduce patient risk is still present (Miller, Steinmetz Pater, & Corman, 2015). In a similar study, computers used to evaluate adverse drug interactions or overdoses in patients did help to decrease adverse events (Rommers, Teepe-Twiss, & Guchelaar, 2011). On the issue of drug development, the Andrew Furman, the co-founder and CEO of 1QBit, states, “Among the likely applications (using quantum computing) is drug development — if you have a completely new molecule and want to predict what effect it will have on the body, one way to do so is to compare it to a database of molecules with known effects. Is it more similar to a molecule that’s known to cure cancer or to cause heart disease?” (Fursman, 2015).
However, some reports are less enthusiastic about CDSSs and quantum learning. The main obstacle with using these machines is the cost. D-wave, a very powerful quantum computer, sells for about $10 million, which is a hefty price for medical research laboratories. Yet, cost in relation to the possibility of a cure for cancer or other disease process should not be an issue, and will likely not be much of an issue as quantum computing becomes more mainstream. In fact, it was recently reported that Europe is planning to spend €1 Billion to develop quantum technology as part of research and development in many areas including medicine(Hellemans, 2016). Many concerns regarding quantum machine learning are the same as those raised regarding CDSS and classical machine learning. Some of these problems include; acceptance by all users, integration into working environments, compatibility with current data management systems, and the ability to upgrade the system as needed (Garg et al., 2005). And, although these systems should reduce medical errors, there are ethical and legal issues to consider before they should be implemented in hospital or laboratory settings. It is also important to understand that this highly sophisticated technology can produce unexpected errors that should be addressed promptly. And, it is also possible that clinicians may become dependent on reminders generated by CDSSs, which could become problematic if a reminder is missed or not given (Mendez Boo et al., 2016; Wang & Summers, 2012). When medical errors occur, it may be difficult to distinguish between a system failure and negligence (Bonney, 2013). Systems that are unable to explain their diagnostic reasoning have primarily been the barrier to implementation within a clinical setting, a problem that quantum computing is unable to solve and may worsen due to the increasing amount of uncertainty.
Finally, given the valid concerns regarding privacy and Healthcare Information Technology, it is imperative that protections be put in place to create an environment that benefits the vendors, patients, and clinicians. Constant breaches of privacy in healthcare have had a significant effect on health care research, significantly slowing the ability to acquire data that allows for rapid and great advances in medicine; advances that help lower healthcare costs and allow the system to pay for itself. Quantum computing does differ slightly when compared to classical learning in that the memory capability of quantum computers can preserve superpositions, which allows the computers to comb through data and find patterns without having to look at individual records.
Although development of smaller and more precise quantum computers are possibly years away from being used, research funding should be allocated to make these computing systems a reality for improvement in healthcare. Integrating the abundance of electronic medical data and quantum machine learning is transforming healthcare, from powering personalized treatments for patients based on their genetic profile to identifying rare disorders that were missed by clinicians through the ability to analyze massive amounts of medical literature and clinical databases that no human is capable of doing. Knowing the sheer power of quantum learning machines makes failing to support their development a detriment to patients, doctors and hospital administrative costs.